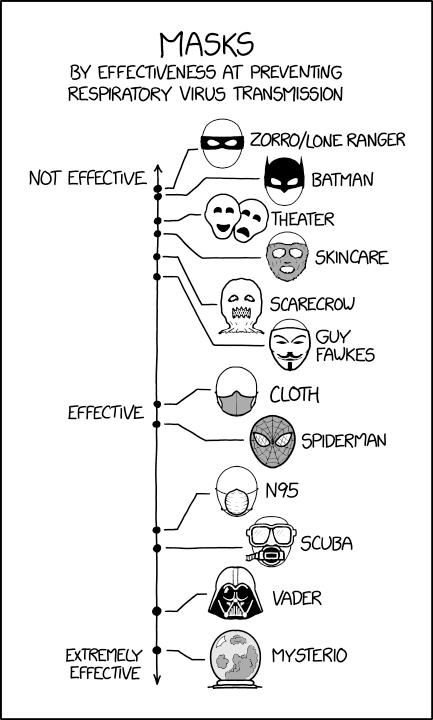
A blog about how-to, internet, social-networks, windows, linux, blogging, tips and tricks.
02 October 2020
Racism thrives on silence -- speak up! | Dexter Dias
Racism thrives on your silence and apathy, says human rights lawyer Dexter Dias. Telling the story of a harrowing UK court case that spotlights the corrosive effects of injustice, Dias urges us all to speak out and expose toxic myths about race -- in order to allow hope, change and justice to flourish.
https://ift.tt/30q9j28
Click this link to view the TED Talk
How to Embed Barcode and QR Code In Google Forms Notifications
The Google Form Notifications add-on lets you automatically send Google Form responses in an email message to one or more recipients. The email notifications are dispatched the moment your form receives a new submission.
The more recent version of the Google Form addon includes support for QR Code and Barcode symbols that you can embed in the email messages. The images can be generated from static text or you can create dynamic images based on answers submitted by the user.
Add QR Code images in Emails
The basic syntax for adding QR Code images in emails is:
=QRCODE(Your Text Goes Here)
You can customize the colors and size of the QR code image by adding key-value pairs in the QRCODE
function.
For instance, if you would like the QRCode to have Indigo background and the QRCode should be itself in white color, the modified formula with the hex color codes would be:
=QRCODE(TEXT textcolor=#FFFFFF backgroundcolor=#4B0082)
The QR Code images have a default width of 300px but if you customize the size with the width
parameter as shown below:
=QRCODE(TEXT width=225)
Include dynamic text in QR Code images
Until now, we have seen examples of static text but the Google Forms addon can also create QR Code images from text in Google Form Answers using placeholders.
For instance, if your Google has a question title “What is your website address?”, you can use a QR Code function like the one below. This will turn the user’s answer into a dynamic QR code that, on scanning, will take you to the form respondent’s website.
=QRCODE({{WWhat is your website address}} textcolor=#4B0082)
Embed Barcode in Email Notifications
The Email Notifications add-on also includes support for BARCODE
function to help you embed barcode images in PNG format for EAN, UPC, ISBN, postal codes, GS1 Database and all other popular formats.
The basic syntax for barcode function is:
=BARCODE(TEXT format=CODE39)
For instance, if you would like to embed the barcode image for a book whose ISBN-13 code is 9781786330895, the function would be:
=BARCODE(9781786330895 format=EAN13 includetext=true)
The includetext=true
parameter would ensure that the text for data is included into the barcode image.
You can also modify the colors of barcode image using hex codes:
=BARCODE(9781786330895 format=EAN13 includetext=true barcolor=AA0000 textcolor=008888 backgroundcolor=FFFF60)
You can similarly modify the width of the Barcode image, the height of the bars, add borders, padding and more. Please consult the documentation for a complete range of formats and options supported by the =BARCODE()
function in Google Forms.
Twitter may let users choose how to crop image previews after bias scrutiny
In an interesting development in the wake of a bias controversy over its cropping algorithm Twitter has said it’s considering giving users decision making power over how tweet previews look, saying it wants to decrease its reliance on machine learning-based image cropping.
Yes, you read that right. A tech company is affirming that automating certain decisions may not, in fact, be the smart thing to do — tacitly acknowledging that removing human agency can generate harm.
As we reported last month, the microblogging platform found its image cropping algorithm garnering critical attention after Ph.D. student Colin Madland noticed the algorithm only showed his own (white male) image in preview — repeatedly cropping out the image of a black faculty member.
Ironically enough he’d been discussing a similar bias issue with Zoom’s virtual backgrounds.
Geez…any guesses why @Twitter defaulted to show only the right side of the picture on mobile? pic.twitter.com/UYL7N3XG9k
— Colin Madland (@colinmadland) September 19, 2020
Twitter responded to the criticism at the time by saying it had tested for bias before shipping the machine learning model and had “not found evidence of racial or gender bias”. But it added: “It’s clear from these examples that we’ve got more analysis to do. We’ll continue to share what we learn, what actions we take, and will open source our analysis so others can review and replicate.”
It’s now followed up with additional details about its testing processes in a blog post where it suggests it could move away from using an algorithm for preview crops in the future.
Twitter also concedes it should have published details of its bias testing process before launching the algorithmic cropping tool — in order that its processes could have been externally interrogated. “This was an oversight,” it admits.
We saw your Tweets about the harm caused by how images are cropped on Twitter.
Today we’re sharing how we test for bias in our systems, and how we plan to rely less on auto-cropping and give you more choice in how images appear in Tweets:
https://t.co/tiSreeoGOA— Twitter Support (@TwitterSupport) October 1, 2020
Explaining how the model works, Twitter writes: “The image cropping system relies on saliency, which predicts where people might look first. For our initial bias analysis, we tested pairwise preference between two demographic groups (White-Black, White-Indian, White-Asian and male-female). In each trial, we combined two faces into the same image, with their order randomized, then computed the saliency map over the combined image. Then, we located the maximum of the saliency map, and recorded which demographic category it landed on. We repeated this 200 times for each pair of demographic categories and evaluated the frequency of preferring one over the other.”
“While our analyses to date haven’t shown racial or gender bias, we recognize that the way we automatically crop photos means there is a potential for harm. We should’ve done a better job of anticipating this possibility when we were first designing and building this product. We are currently conducting additional analysis to add further rigor to our testing, are committed to sharing our findings, and are exploring ways to open-source our analysis so that others can help keep us accountable,” it adds.
On the possibility of moving away from algorithmic image cropping in favor of letting humans have a say, Twitter says it’s “started exploring different options to see what will work best across the wide range of images people tweet every day”.
“We hope that giving people more choices for image cropping and previewing what they’ll look like in the tweet composer may help reduce the risk of harm,” it adds, suggesting tweet previews could in future include visual controls for users.
Such a move, rather than injecting ‘friction’ into the platform (which would presumably be the typical techie concern about adding another step to the tweeting process), could open up new creative/tonal possibilities for Twitter users by providing another layer of nuance that wraps around tweets. Say by enabling users to create ‘easter egg’ previews that deliberately conceal a key visual detail until someone clicks through; or which zero-in on a particular element to emphasize a point in the tweet.
Given the popularity of joke ‘half and half’ images that play with messaging app WhatsApp’s preview crop format — which requires a click to predictably expand the view — it’s easy to see similar visual jokes and memes being fired up on Twitter, should it provide users with the right tools.
The bottom line is that giving humans more agency means you’re inviting creativity — and letting diversity override bias. Which should be a win-win. So it’s great to see Twitter entertaining the idea of furloughing one of its algorithms. (Dare we suggest the platform also takes a close and critical look at the algorithmic workings around ‘top tweets’, ‘trending tweets’, and the ‘popular/relevant’ content its algos sometimes choose to inject, unasked, into users’ timelines, all of which can generate a smorgasbord of harms.)
Returning to image cropping, Twitter says that as a general rule it will be committed to “the ‘what you see is what you get’ principles of design” — aka, “the photo you see in the tweet composer is what it will look like in the tweet” — while warning there will likely still be some exceptions, such as for images that aren’t a standard size.
In those cases it says it will experiment with how such images are presented, aiming to do so in a way that “doesn’t lose the creator’s intended focal point or take away from the integrity of the photo”. Again, it will do well to show any algorithmic workings in public.
Read Full Article
Facebook sues two companies engaged in data scraping operations
Facebook today says it has filed a lawsuit in the U.S. against two companies that had engaged in an international “data scraping” operation. The operation extended across Facebook properties, including both Facebook and Instagram, as well as other large websites and services, including Twitter, Amazon, LinkedIn and YouTube. The companies, which gathered the data of Facebook users for “marketing intelligence” purposes, did so in violation of Facebook’s Terms of Service, says Facebook.
The businesses named in the lawsuit are Israeli-based BrandTotal Ltd. and Unimania Inc., a business incorporated in Delaware.
According to BrandTotal’s website, its company offers a real-time competitive intelligence platform that’s designed to give media, insights and analytics teams visibility into their competition’s social media strategy and paid campaigns. These insights would allow its customers to analyze and shift their budget allocation to target new opportunities, monitor trends and threats from emerging brands, optimize their ads and messaging and more.
Meanwhile, Unimania operated apps claimed to offer users the ability to access social networks in different ways. For example, Unimania offered apps that let you view Facebook via a mobile-web interface or alongside other social networks like Twitter. Another app let you view Instagram Stories anonymously, it claimed.
However, Facebook’s lawsuit is largely focused on two browser extensions offered by the companies: Unimania’s “Ads Feed” and BrandTotal’s “UpVoice.”
The former allowed users to save the ads they saw on Facebook for later reference. But as the extension’s page discloses, doing so would opt users into a panel that informed the advertising decisions of Unimania’s corporate customers. UpVote, on the other hand, rewarded users with gift cards for using top social networking and shopping sites and sharing their opinions about the online campaigns run by big brands.
Facebook says these extensions operated in violation of its protections against scraping and its terms of service. When users installed the extensions and visited Facebook websites, the extensions installed automated programs to scrape their name, user ID, gender, date of birth, relationship status, location information and other information related to their accounts. The data was then sent to a server shared by BrandTotal and Unimania.
Facebook lawsuit vs BrandTotal Ltd. and Unimania Inc. by TechCrunch on Scribd
Data scrapers exist in part to collect as much information as they can through any means possible using automated tools, like bots and scripts. Cambridge Analytica infamously scraped millions of Facebook profiles in the run-up to the 2016 presidential election in order to target undecided voters. Other data scraping operations use bots to monitor concert or event ticket prices in order to undercut competitors. Scraped data can also be used for marketing and advertising, or simply sold on to others.
In the wake of the Cambridge Analytica scandal, Facebook has begun to pursue legal action against various developers that break its terms of service.
Most cases involving data scraping are litigated under the Computer Fraud and Abuse Act, written in the 1980s to prosecute computer hacking cases. Anyone who accesses a computer “without authorization” can face hefty fines or even prison time.
But because the law doesn’t specifically define what “authorized” access is and what isn’t, tech giants have seen mixed results in their efforts to shut down data scrapers.
LinkedIn lost its high-profile case against HiQ Labs in 2019 after an appeals court ruled that the scraper was only collecting data that was publicly available from the internet. Internet rights groups like the Electronic Frontier Foundation lauded the decision, arguing that internet users should not face legal threats “simply for accessing publicly available information in a way that publishers object to.”
Facebook’s latest legal case is slightly different because the company is accusing BrandTotal of scraping Facebook profile data that wasn’t inherently public. Facebook says the accused data scraper used a browser extension installed on users’ computers to gain access to their Facebook profile data.
In March 2019, it took action against two Ukrainian developers who were harvesting data using quiz apps and browser extensions to scrape profile information and people’s friends lists, Facebook says. A court in California recently recommended a judgement in Facebook’s favor in the case. A separate case around scraping filed last year against a marketing partner, Stackla, also came back in Facebook’s favor.
This year, Facebook filed lawsuits against companies and individuals engaged in both scraping and fake engagement services.
Facebook isn’t just cracking down on data scraping businesses to protect user privacy, however. It’s because failing to do so can lead to large fines. Facebook at the beginning of this year was ordered to pay out over half a billion dollars to settle a class action lawsuit that alleged systemic violation of an Illinois privacy law. Last year, it settled with the FTC over privacy lapses and had to pay a $5 billion penalty. As governments work to further regulation of online privacy and data violations, fines like this could add up.
The company says legal action isn’t the only way it’s working to stop data scraping. It has also invested in technical teams and tools to monitor and detect suspicious activity and the use of unauthorized automation for scraping, it says.
Read Full Article
Facebook won’t accept ads that ‘delegitimize’ US election results
Following a particularly dark and vivid display of the threats to the 2020 U.S. election during Tuesday’s first presidential debate, Facebook has further clarified its new rules around election-related ads.
Facebook is now expanding its political advertising rules to disallow any ads that “[seek] to delegitimize the outcome of an election” including “calling a method of voting inherently fraudulent or corrupt, or using isolated incidents of voter fraud to delegitimize the result of an election.”
Facebook Director of Product Management Rob Leathern, who leads the company’s business integrity team, announced the changes on Twitter.
For example, this would include calling a method of voting inherently fraudulent or corrupt, or using isolated incidents of voter fraud to delegitimize the result of an election. You can find more info and specifics in our Help Center here https://t.co/BPnm1z7LW6 (2/3)
— Rob Leathern (@robleathern) September 30, 2020
Facebook says it will also not allow ads that discourage users from voting, undermine vote-by-mail or other lawful voting methods, suggest voter fraud is widespread, threaten safe voting through false health claims and ads that suggest the vote is invalid because results might not be immediately known on election night.
Both Twitter and Facebook recently issued new guidelines on how they will handle claims of election victory prior to official results, though Facebook’s rules appear to only apply to those claims if they’re made in advertising. We’ve asked Facebook for clarification about how those claims will be handled outside of ads, on a candidate’s normal account.
While Twitter opted to no longer accept political advertising across the board, Facebook is instead tweaking its rules about what kinds of political ads it will allow and when. Facebook previously announced that it would no longer accept ads about elections, social issues or politics in the U.S. after October 27, though political ads that ran before that date will be allowed to continue.
Facebook is already grappling with a deluge of attacks on the integrity of November’s U.S. election originating with President Trump and his supporters. During Tuesday night’s debate, Trump again cast doubt about voting by mail (a system already trusted and in use nationwide in the form of absentee ballots) and declined to commit to accepting election results if he loses.
While the unique circumstances of the pandemic are leading to logistical challenges, voting through the mail is not new. A handful of states, including Colorado and Oregon, already conducted elections through the mail, and vote-by-mail is just a scaled-up version of the absentee voting systems already in place nationwide.
On Wednesday, President Trump sowed conspiratorial ideas about defective ballots that were sent out in New York state as a result of vendor error. The state will reissue the ballots, but Trump seized on the incident as evidence that vote-by-mail is a “scam” — a claim that evidence does not bear out.
Trump’s attacks on the U.S. election are an unprecedented challenge for social platforms, but also one for the nation as a whole, which has never in modern times seen the peaceful transfer of executive power threatened by a sitting president.
Read Full Article
Daily Crunch: Google commits $1B to pay publishers
Google is paying a lot of money for its news licensing program, Microsoft announces an affordable laptop and Facebook says it won’t accept ads casting doubts on the election. This is your Daily Crunch for October 1, 2020.
The big story: Google commits $1B to pay publishers
Specifically, CEO Sundar Pichai said today that the company will be paying $1 billion to news publishers to license their content for a new format called the Google News Showcase — basically, panels highlighting stories from partner publishers in Google News.
Google outlined the broad strokes of this plan over the summer, but now it’s actually launching, and it has signed deals with 200 publications in Germany, Brazil, Argentina, Canada, the U.K. and Australia.
This announcement also comes as Google and Facebook are both facing battles in a number of countries as regulators and publishers pressure them for payments.
The tech giants
Microsoft adds the $549 Laptop Go to its growing Surface lineup — At $549, the Laptop Go is $50 more than the Surface Go tablet, but it’s still an extremely affordable take on the category.
Facebook won’t accept ads that ‘delegitimize’ US election results — Facebook said this includes ads “calling a method of voting inherently fraudulent or corrupt, or using isolated incidents of voter fraud to delegitimize the result of an election.”
Google now has three mid-range Pixel phones — Brian Heater unpacks the company’s smartphone strategy.
Startups, funding and venture capital
Working for social justice isn’t a ‘distraction’ for mission-focused companies — Passion Capital’s Eileen Burbidge weighs in on Coinbase’s controversial stance on politics.
Cazoo, the UK used car sales platform, raises another $311M, now valued at over $2.5B — The funding comes only six months after the company raised $116 million.
With $18M in new funding, Braintrust says it’s creating a fairer model for freelancers — The startup is using a cryptocurrency token that it calls Btrust to reward users who build the network.
Advice and analysis from Extra Crunch
Latin America’s digital transformation is making up for lost time — After more than a decade of gradual progress made through fits and starts, tech in Latin America finally hit its stride.
News apps in the US and China use algorithms to drive engagement, discovery — We examine various players in the field and ask how their black boxes affect people’s content consumption.
(Reminder: Extra Crunch is our subscription membership program, which aims to democratize information about startups. You can sign up here.)
Everything else
Section 230 will be on the chopping block at the next big tech hearing — It looks like we’re in for another big tech CEO hearing.
What if the kernel is corrupt? — The latest episode of Equity discusses moderation issues at Clubhouse.
The Daily Crunch is TechCrunch’s roundup of our biggest and most important stories. If you’d like to get this delivered to your inbox every day at around 3pm Pacific, you can subscribe here.
Read Full Article
Google takes aim at ‘beauty filters’ with design changes coming to Pixel phones
Google is taking aim at photo face filters and other “beautifying” techniques that mental health experts believe can warp a person’s self-confidence, particularly when they’re introduced to younger users. The company says it will now rely on expert guidance when applying design principles for photos filters used by the Android Camera app on Pixel smartphones. In the Pixel 4a, Google has already turned off face retouching by default, it says, and notes the interface will soon be updated to include what Google describes as “value-free” descriptive icons and labels for the app’s face retouching effects.
That means it won’t use language like “beauty filter” or imply, even in more subtle ways, that face retouching tools can make someone look better. These changes will also roll out to the Android Camera app in other Pixel smartphones through updates.
The changes, though perhaps unnoticed by the end user, can make a difference over time.
Google says that over 70% of photos on Android are shot with the front-facing camera and over 24 billion photos have been labeled as “selfies” in Google Photos.
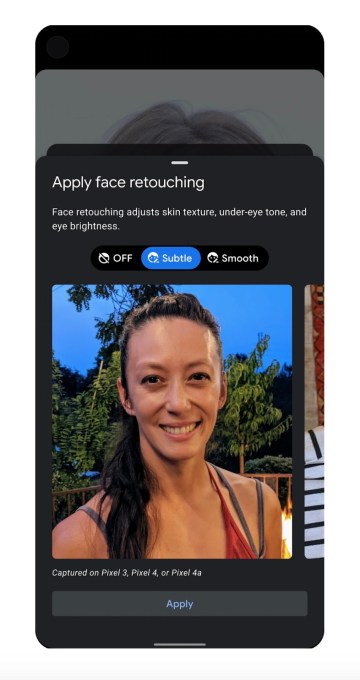
Image Credits: Google
But the images our smartphones are showing us are driving more people to be dissatisfied with their own apparences. According to the American Academy of Facial Plastic and Reconstructive Surgery, 72% of their members last year said their patients had sought them out in order to improve their selfies, a 15% year-over-year increase. In addition, 80% of parents said they’re worried about filters’ impact and two-thirds of teens said they’ve been bullied over how they look in photos.
Google explains it sought the help of child and mental health experts to better understand the impact of filters on people’s well-being. It found that when people weren’t aware a photo filter had been applied, the resulting photos could negatively impact mental well-being as they quietly set a beauty standard that people would then compare themselves against over time.
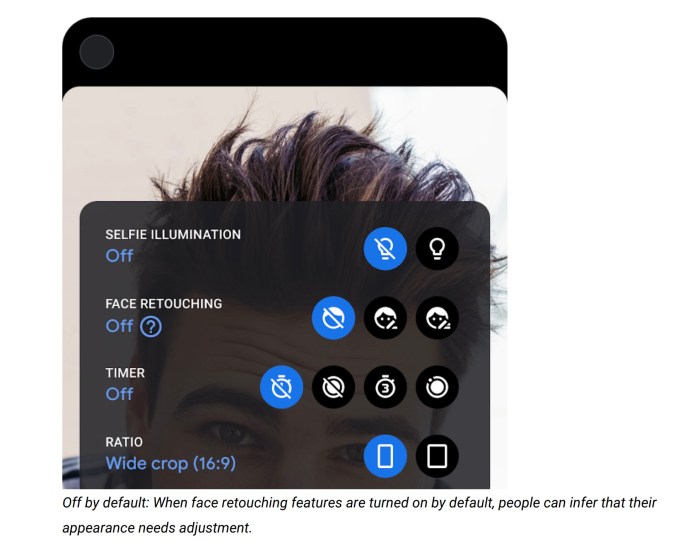
Image Credits: Google
In addition, filters that use terminology like “beauty,” “beautification,” “enhancement,” and “touch up” imply there’s something wrong with someone’s physical appearance that needs to be corrected. It suggests that the way they actually look is bad, Google explains. The same is true for terms like “slimming” which imply a person’s body needs to be improved.
Google also found that even the icons used could contribute to the problem.
It’s often the case that face retouching filters will use “sparkling” design elements on the icon that switches the feature on. This suggests that using the filter is making your photo better.
To address this problem, Google will update to using value-neutral language for its filters along with new icons.
For example, instead of labeling a face retouching option as “natural,” it will relabel it to “subtle.” And instead of sparkling icons, it instead shows an icon of the face with an editing pen to indicate which button to push to enable the feature.
Adjustment levels will also follow new guidelines, and use either numbers and symbols or simple terms like “low” and “high,” rather than those that refer to beauty.
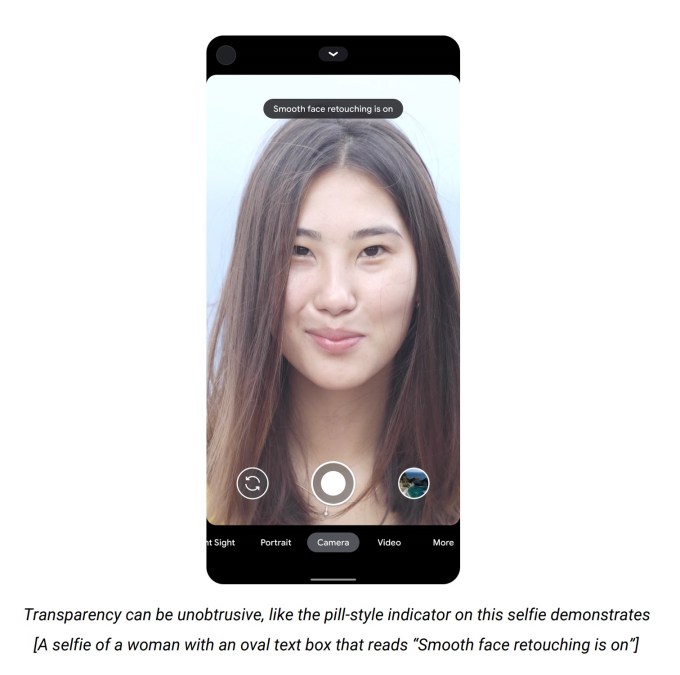
Image Credits: Google
Google says the Camera app, too, should also make it obvious when a filter has been enabled — both in the real-time capture and afterwards. For example, an indicator at the top of the screen could inform the user when a filter has been turned on, so users know their image is being edited.
In Pixel smartphones, starting with the Pixel 4a, when you use face retouching effects, you’ll be shown more information about how each setting is being applied and what specific changes it will make to the image. For instance, if you choose the “subtle” effect, it will explain that it adjusts your skin texture, under eye tone, and eye brightness. Being transparent about the effects applied can help to demystify the sometimes subtle tweaks that face retouching filters are making to our photos.
Face retouching will also be shut off in the new Pixel devices announced on Wednesday, including the Pixel 4a 5G and Pixel 5. And the changes to labels and descriptions are coming to Pixel phones through an upcoming update, Google says, which will support Pixel 2 and later devices.
Read Full Article
Developing Real-Time, Automatic Sign Language Detection for Video Conferencing
Posted by Amit Moryossef, Research Intern, Google Research
Video conferencing should be accessible to everyone, including users who communicate using sign language. However, since most video conference applications transition window focus to those who speak aloud, it makes it difficult for signers to “get the floor” so they can communicate easily and effectively. Enabling real-time sign language detection in video conferencing is challenging, since applications need to perform classification using the high-volume video feed as the input, which makes the task computationally heavy. In part, due to these challenges, there is only limited research on sign language detection.
In “Real-Time Sign Language Detection using Human Pose Estimation”, presented at SLRTP2020 and demoed at ECCV2020, we present a real-time sign language detection model and demonstrate how it can be used to provide video conferencing systems a mechanism to identify the person signing as the active speaker.
![]() |
Maayan Gazuli, an Israeli Sign Language interpreter, demonstrates the sign language detection system. |
Our Model
To enable a real-time working solution for a variety of video conferencing applications, we needed to design a light weight model that would be simple to “plug and play.” Previous attempts to integrate models for video conferencing applications on the client side demonstrated the importance of a light-weight model that consumes fewer CPU cycles in order to minimize the effect on call quality. To reduce the input dimensionality, we isolated the information the model needs from the video in order to perform the classification of every frame.
Because sign language involves the user’s body and hands, we start by running a pose estimation model, PoseNet. This reduces the input considerably from an entire HD image to a small set of landmarks on the user’s body, including the eyes, nose, shoulders, hands, etc. We use these landmarks to calculate the frame-to-frame optical flow, which quantifies user motion for use by the model without retaining user-specific information. Each pose is normalized by the width of the person’s shoulders in order to ensure that the model attends to the person signing over a range of distances from the camera. The optical flow is then normalized by the video’s frame rate before being passed to the model.
To test this approach, we used the German Sign Language corpus (DGS), which contains long videos of people signing, and includes span annotations that indicate in which frames signing is taking place. As a naïve baseline, we trained a linear regression model to predict when a person is signing using optical flow data. This baseline reached around 80% accuracy, using only ~3μs (0.000003 seconds) of processing time per frame. By including the 50 previous frames’ optical flow as context to the linear model, it is able to reach 83.4%.
To generalize the use of context, we used a long-short-term memory (LSTM) architecture, which contains memory over the previous timesteps, but no lookback. Using a single layer LSTM, followed by a linear layer, the model achieves up to 91.5% accuracy, with 3.5ms (0.0035 seconds) of processing time per frame.
![]() |
Classification model architecture. (1) Extract poses from each frame; (2) calculate the optical flow from every two consecutive frames; (3) feed through an LSTM; and (4) classify class. |
Proof of Concept
Once we had a functioning sign language detection model, we needed to devise a way to use it for triggering the active speaker function in video conferencing applications. We developed a lightweight, real-time, sign language detection web demo that connects to various video conferencing applications and can set the user as the “speaker” when they sign. This demo leverages PoseNet fast human pose estimation and sign language detection models running in the browser using tf.js, which enables it to work reliably in real-time.
When the sign language detection model determines that a user is signing, it passes an ultrasonic audio tone through a virtual audio cable, which can be detected by any video conferencing application as if the signing user is “speaking.” The audio is transmitted at 20kHz, which is normally outside the hearing range for humans. Because video conferencing applications usually detect the audio “volume” as talking rather than only detecting speech, this fools the application into thinking the user is speaking.
![]() |
The sign language detection demo takes the webcam’s video feed as input, and transmits audio through a virtual microphone when it detects that the user is signing. |
You can try our experimental demo right now! By default, the demo acts as a sign language detector. The training code and models as well as the web demo source code is available on GitHub.
Demo
In the following video, we demonstrate how the model might be used. Notice the yellow chart at the top left corner, which reflects the model’s confidence in detecting that activity is indeed sign language. When the user signs, the chart values rise to nearly 100, and when she stops signing, it falls to zero. This process happens in real-time, at 30 frames per second, the maximum frame rate of the camera used.
Maayan Gazuli, an Israeli Sign Language interpreter, demonstrates the sign language detection demo. |
User Feedback
To better understand how well the demo works in practice, we conducted a user experience study in which participants were asked to use our experimental demo during a video conference and to communicate via sign language as usual. They were also asked to sign over each other, and over speaking participants to test the speaker switching behavior. Participants responded positively that sign language was being detected and treated as audible speech, and that the demo successfully identified the signing attendee and triggered the conferencing system’s audio meter icon to draw focus to the signing attendee.
Conclusions
We believe video conferencing applications should be accessible to everyone and hope this work is a meaningful step in this direction. We have demonstrated how our model could be leveraged to empower signers to use video conferencing more conveniently.
Acknowledgements
Amit Moryossef, Ioannis Tsochantaridis, Roee Aharoni, Sarah Ebling, Annette Rios, Srini Narayanan, George Sung, Jonathan Baccash, Aidan Bryant, Pavithra Ramasamy and Maayan Gazuli
Prosus Ventures leads $13 million investment in Pakistan’s ride-hailing giant Bykea
Bykea, which leads the ride-hailing market in Pakistan, has raised $13 million in a new financing round as the five-year-old startup looks to deepen its penetration in the South Asian country and become a “super app.”
The startup’s new financing round, a Series B, was led by storied investment firm Prosus Ventures. It’s the first time Prosus Ventures has invested in a Pakistani startup. Bykea’s existing investors Middle East Venture Partners and Sarmayacar also invested in the round, which brings its total to-date raise to $22 million.
Bykea leads the two-wheeler ride-hailing market in Pakistan, and also operates a logistics delivery business and a financial services business. The startup has partnered with banks to allow customers to pay phone bills and get cash delivered to them, Muneeb Maayr, founder and chief executive of Bykea, told TechCrunch in an interview.
Fahd Beg, chief investment officer at Prosus, said firms like Bykea are helping transform big societal needs like transportation, logistics and payments through a technology-enabled platform in Pakistan. “Bykea has already seen impressive traction in the country and with our investment will be able to execute further on their vision to become Pakistan’s ‘super app,’ ” he said in a statement.
Bykea works with more than 30,000 drivers who operate in Karachi, Rawalpindi and Lahore. (Two-wheelers are more popular in Pakistan. There are about 17 million two-wheeler vehicles on the road in the country today, compared to fewer than 4 million cars.)
The new investment comes at a time when Bykea restores the losses incurred by the coronavirus outbreak. Like several nations, Pakistan enforced a months-long lockdown to curtail the spread of the virus in March.
As with most other startups in travel business globally, this meant bad news for Bykea. Maayr said the startup did not eliminate jobs and instead cut several other expenses to navigate the tough time.
One of those cuts was curtailing the startup’s reliance on Google Maps. Maayr said during the lockdown Bykea built its own mapping navigation system with the help of its drivers. The startup, which was paying Google about $60,000 a month for using Maps, now pays less than a tenth of it, he said.
Starting in August, the startup’s operations have largely recovered, and it is looking to further expand its financial services business, said Maayr, who previously worked for Rocket Internet, helping the giant run fashion e-commerce platform Daraz in the country.
The startup has been able to out-compete firms like Careem and Uber in Pakistan by offering localized solutions. It remains one of the few internet businesses in the country that supports the Urdu language in its app, for instance.
“Our brand is now widely used as a verb for bike taxi and 30-minute deliveries, and the fresh capital allows us to expand our network to solidify our leading position,” he said.
I asked Maayr what he thinks of the opportunities in the three-wheelers category. Auto-rickshaws are some of the most popular mode of transportation in South Asian nations. Maayr said on-boarding those drivers and figuring out unit-economics that works for all the stakeholders remains a challenge in all South Asian nations, so the startup is still figuring it out.
Would he want to take Bykea to neighboring nations? Not anytime soon. Maayr said the opportunity within Pakistan and Bykea’s traction in the nation have convinced him to win the entire local market first.
Read Full Article
Audiovisual Speech Enhancement in YouTube Stories
Posted by Inbar Mosseri, Software Engineer and Michael Rubinstein, Research Scientist, Google Research
While tremendous efforts are invested in improving the quality of videos taken with smartphone cameras, the quality of audio in videos is often overlooked. For example, the speech of a subject in a video where there are multiple people speaking or where there is high background noise might be muddled, distorted, or difficult to understand. In an effort to address this, two years ago we introduced Looking to Listen, a machine learning (ML) technology that uses both visual and audio cues to isolate the speech of a video’s subject. By training the model on a large-scale collection of online videos, we are able to capture correlations between speech and visual signals such as mouth movements and facial expressions, which can then be used to separate the speech of one person in a video from another, or to separate speech from background sounds. We showed that this technology not only achieves state-of-the-art results in speech separation and enhancement (a noticeable 1.5dB improvement over audio-only models), but in particular can improve the results over audio-only processing when there are multiple people speaking, as the visual cues in the video help determine who is saying what.
We are now happy to make the Looking to Listen technology available to users through a new audiovisual Speech Enhancement feature in YouTube Stories (on iOS), allowing creators to take better selfie videos by automatically enhancing their voices and reducing background noise. Getting this technology into users’ hands was no easy feat. Over the past year, we worked closely with users to learn how they would like to use such a feature, in what scenarios, and what balance of speech and background sounds they would like to have in their videos. We heavily optimized the Looking to Listen model to make it run efficiently on mobile devices, overall reducing the running time from 10x real-time on a desktop when our paper came out, to 0.5x real-time performance on the phone. We also put the technology through extensive testing to verify that it performs consistently across different recording conditions and for people with different appearances and voices.
From Research to Product
Optimizing Looking to Listen to allow fast and robust operation on mobile devices required us to overcome a number of challenges. First, all processing needed to be done on-device within the client app in order to minimize processing time and to preserve the user’s privacy; no audio or video information would be sent to servers for processing. Further, the model needed to co-exist alongside other ML algorithms used in the YouTube app in addition to the resource-consuming video recording itself. Finally, the algorithm needed to run quickly and efficiently on-device while minimizing battery consumption.
The first step in the Looking to Listen pipeline is to isolate thumbnail images that contain the faces of the speakers from the video stream. By leveraging MediaPipe BlazeFace with GPU accelerated inference, this step is now able to be executed in just a few milliseconds. We then switched the model part that processes each thumbnail separately to a lighter weight MobileNet (v2) architecture, which outputs visual features learned for the purpose of speech enhancement, extracted from the face thumbnails in 10 ms per frame. Because the compute time to embed the visual features is short, it can be done while the video is still being recorded. This avoids the need to keep the frames in memory for further processing, thereby reducing the overall memory footprint. Then, after the video finishes recording, the audio and the computed visual features are streamed to the audio-visual speech separation model which produces the isolated and enhanced speech.
We reduced the total number of parameters in the audio-visual model by replacing “regular” 2D convolutions with separable ones (1D in the frequency dimension, followed by 1D in the time dimension) with fewer filters. We then optimized the model further using TensorFlow Lite — a set of tools that enable running TensorFlow models on mobile devices with low latency and a small binary size. Finally, we reimplemented the model within the Learn2Compress framework in order to take advantage of built-in quantized training and QRNN support.
![]() |
Our Looking to Listen on-device pipeline for audiovisual speech enhancement |
These optimizations and improvements reduced the running time from 10x real-time on a desktop using the original formulation of Looking to Listen, to 0.5x real-time performance using only an iPhone CPU; and brought the model size down from 120MB to 6MB now, which makes it easier to deploy. Since YouTube Stories videos are short — limited to 15 seconds — the result of the video processing is available within a couple of seconds after the recording is finished.
Finally, to avoid processing videos with clean speech (so as to avoid unnecessary computation), we first run our model only on the first two seconds of the video, then compare the speech-enhanced output to the original input audio. If there is sufficient difference (meaning the model cleaned up the speech), then we enhance the speech throughout the rest of the video.
Researching User Needs
Early versions of Looking to Listen were designed to entirely isolate speech from the background noise. In a user study conducted together with YouTube, we found that users prefer to leave in some of the background sounds to give context and to retain some the general ambiance of the scene. Based on this user study, we take a linear combination of the original audio and our produced clean speech channel: output_audio = 0.1 x original_audio + 0.9 x speech. The following video presents clean speech combined with different levels of the background sounds in the scene (10% background is the balance we use in practice).
Below are additional examples of the enhanced speech results from the new Speech Enhancement feature in YouTube Stories. We recommend watching the videos with good speakers or headphones.
Fairness Analysis
Another important requirement is that the model be fair and inclusive. It must be able to handle different types of voices, languages and accents, as well as different visual appearances. To this end, we conducted a series of tests exploring the performance of the model with respect to various visual and speech/auditory attributes: the speaker’s age, skin tone, spoken language, voice pitch, visibility of the speaker’s face (% of video in which the speaker is in frame), head pose throughout the video, facial hair, presence of glasses, and the level of background noise in the (input) video.
For each of the above visual/auditory attributes, we ran our model on segments from our evaluation set (separate from the training set) and measured the speech enhancement accuracy, broken down according to the different attribute values. Results for some of the attributes are summarized in the following plots. Each data point in the plots represents hundreds (in most cases thousands) of videos fitting the criteria.
![]() |
Left: Speech enhancement quality as a function of the speaker’s voice pitch. The fundamental voice frequency (pitch) of an adult male typically ranges from 85 to 180 Hz, and that of an adult female ranges from 165 to 255 Hz. Right: speech enhancement quality as a function of the speaker’s predicted age. |
Using the Feature
YouTube creators who are eligible for YouTube Stories creation may record a video on iOS, and select “Enhance speech” from the volume controls editing tool. This will immediately apply speech enhancement to the audio track and will play back the enhanced speech in a loop. It is then possible to toggle the feature on and off multiple times to compare the enhanced speech with the original audio.
In parallel to this new feature in YouTube, we are also exploring additional venues for this technology. More to come later this year — stay tuned!
Acknowledgements
This feature is a collaboration across multiple teams at Google. Key contributors include: from Research-IL: Oran Lang; from VisCAM: Ariel Ephrat, Mike Krainin, JD Velasquez, Inbar Mosseri, Michael Rubinstein; from Learn2Compress: Arun Kandoor; from MediaPipe: Buck Bourdon, Matsvei Zhdanovich, Matthias Grundmann; from YouTube: Andy Poes, Vadim Lavrusik, Aaron La Lau, Willi Geiger, Simona De Rosa, and Tomer Margolin.