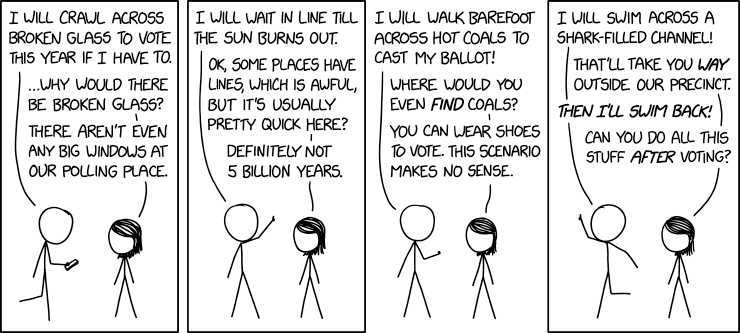
A blog about how-to, internet, social-networks, windows, linux, blogging, tips and tricks.
19 September 2020
Unity Software has strong opening, gaining 31% after pricing above its raised range
Whoever said you can’t make money playing video games clearly hasn’t taken a look at Unity Software’s stock price.
On its first official day of trading, the company rose more than 31%, opening at $75 per share before closing the day at $68.35. Unity’s share price gains came after last night’s pricing of the company’s stock at $52 per share, well above the range of $44 to $48 which was itself an upward revision of the company’s initial target.
Games like “Pokémon GO” and “Iron Man VR” rely on the company’s software, as do untold numbers of other mobile gaming applications that use the company’s toolkit for support. The company’s customers range from small gaming publishers to large gaming giants like Electronic Arts, Niantic, Ubisoft and Tencent.
Unity’s IPO comes on the heels of other well-received debuts, including Sumo Logic, Snowflake and JFrog.
TechCrunch caught up with Unity’s CFO, Kim Jabal, after-hours today to dig in a bit on the transaction.
According to Jabal, hosting her company’s roadshow over Zoom had some advantages, as her team didn’t have to focus on tackling a single geography per day, allowing Unity to “optimize” its time based on who the company wanted to meet, instead, of say, whomever was free in Boston or Chicago on a particular Tuesday morning.
Jabal’s comments aren’t the first that TechCrunch has heard regarding roadshows going well in a digital format instead of as an in-person presentation. If the old-school roadshow survives, we’ll be surprised, though private jet companies will miss the business.
Talking about the transaction itself, Jabal stressed the connection between her company’s employees, value and their access to that same value. Unity’s IPO was unique in that existing and former employees were able to trade 15% of their vested holdings in the company on day one, excluding “current executive officers and directors,” per SEC filings.
That act does not seemed to have dampened enthusiasm for the company’s shares, and could have helped boost early float, allowing for the two sides of the supply and demand curves to more quickly meet close to the company’s real value, instead of a scarcity-driven, more artificial figure.
Regarding Unity’s IPO pricing, Jabal discussed what she called a “very data-driven process.” The result of that process was an IPO price that came in above its raised range, and still rose during its first day’s trading, but less than 50%. That’s about as good an outcome as you can hope for in an IPO.
One final thing for the SaaS nerds out there. Unity’s “dollar-based net expansion rate” went from very good to outstanding in 2020, or in the words of the S-1/A:
Our dollar-based net expansion rate, which measures expansion in existing customers’ revenue over a trailing 12-month period, grew from 124% as of December 31, 2018 to 133% as of December 31, 2019, and from 129% as of June 30, 2019 to 142% as of June 30, 2020, demonstrating the power of this strategy.
We had to ask. And the answer, per Jabal, was a combination of the company’s platform strength and how customers tend to use more of Unity’s services over time, which she described as growing with their customers. And the second key element was 2020’s unique dynamics that gave Unity a “tailwind” thanks to “increased usage, particularly in gaming.”
Looking at our own gaming levels in 2020 compared to 2019, that checks out.
This post closes the book on this week’s IPO class. Tired yet? Don’t be. Palantir is up next, and then Asana.
Read Full Article
Daily Crunch: Partial US TikTok ban is imminent
The Trump administration moves forwards with plans to ban TikTok and WeChat (although TikTok gets a partial extension), Unity goes public and we announce the winner of this year’s Startup Battlefield. This is your Daily Crunch for September 18, 2020.
The big story: US TikTok ban is imminent
The U.S. Commerce Department has released details about how it will be implementing the Trump administration’s domestic ban of TikTok and WeChat. Both apps will no longer be available (and will not be able to distribute updates) in U.S. app stores starting this Sunday, September 20.
At the same time, TikTok will be able to continue operations in the country until November 12, leaving the door open for a deal with Oracle or another partner.
TikTok, WeChat and their users aren’t the only ones unhappy about this decision. Instagram CEO Adam Mosseri said a TikTok ban would be “bad for US tech companies which have benefited greatly from the ability to operate across borders,” while the ACLU said the order “violates the First Amendment rights of people in the United States.”
The tech giants
Salesforce announces 12,000 new jobs in the next year just weeks after laying off 1,000 — Salesforce CEO and co-founder Marc Benioff announced in a tweet that the company would be hiring 4,000 new employees in the next six months, and 12,000 in the next year.
It’s game on as Unity begins trading — Unity Software, which sells a game development toolkit primarily for mobile phone app developers, raised $1.3 billion in its initial public offering.
Apple will launch its online store in India on September 23 — Apple currently relies on third-party online and offline retailers to sell its products in India.
Startups, funding and venture capital
And the winner of Startup Battlefield at Disrupt 2020 is … Canix — After five days of fierce pitching in a wholly new virtual Startup Battlefield arena, we have a winner.
Amid layoffs and allegations of fraud, the FBI has arrested NS8’s CEO following its $100+ million summer financing — Adam Rogas, the co-founder and former executive at the Las Vegas-based fraud prevention company NS8 was arrested by the Federal Bureau of Investigation.
Outschool, newly profitable, raises a $45 million Series B for virtual small group classes — Outschool’s services, which range from engineering lessons through Lego challenges to Spanish teaching by Taylor Swift songs, are now high in demand.
Advice and analysis from Extra Crunch
Are high churn rates depressing earnings for app developers? — RevenueCat’s Jacob Eiting writes that for all the hype around Apple’s 85/15 split for subscription revenue, very few developers are going to see a meaningful increase.
The stages of traditional fundraising — What you think when you hear “seed funding” and “A rounds” might be different from what investors think.
3 VCs discuss the state of SaaS investing in 2020 — Commentary from Canaan’s Maha Ibrahim, Andreessen Horowitz’s David Ulevitch and Bessemer’s Mary D’Onofrio.
(Reminder: Extra Crunch is our subscription membership program, which aims to democratize information about startups. You can sign up here.)
Everything else
How the NSA is disrupting foreign hackers targeting COVID-19 vaccine research — “The threat landscape has changed,” the NSA’s director of cybersecurity Anne Neuberger said at Disrupt 2020.
NASA to test precision automated landing system designed for the moon and Mars on upcoming Blue Origin mission — The “Safe and Precise Landing – Integrated Capabilities Evolution” (SPLICE) system is made up of a number of lasers, an optical camera and a computer.
The Daily Crunch is TechCrunch’s roundup of our biggest and most important stories. If you’d like to get this delivered to your inbox every day at around 3pm Pacific, you can subscribe here.
Read Full Article
Are high churn rates depressing earnings for app developers?
Ever since Apple opened up subscription monetization to more apps in 2016 — and enticed developers with an 85/15 split on revenue from customers that remain subscribed for more than a year — subscription monetization and retention has felt like the Holy Grail for app developers. So much so that Google quickly followed suit in what appeared to be an example of healthy competition for developers in the mobile OS duopoly.
But how does that split actually work out for most apps? Turns out, the 85/15 split — which Apple is keen to mention anytime developers complain about the App Store rev share — doesn’t have a meaningful impact for most developers. Because churn.
No matter how great an app is, subscribers are going to churn. Sometimes it’s because of a credit card expiring or some other billing issue. And sometimes it’s more of a pause, and the user comes back after a few months. But the majority of churn comes from subscribers who, for whatever reason, decide that the app just isn’t worth paying for anymore. If a subscriber churns before the one-year mark, the developer never sees that 85% split. And even if the user resubscribes, Apple and Google reset the clock if a subscription has lapsed for more than 60 days. Rather convenient… for Apple and Google.
Top mobile apps like Netflix and Spotify report churn rates in the low single digits, but they are the outliers. According to our data, the median churn rate for subscription apps is around 13% for monthly subscriptions and around 50% for annual. Monthly subscription churn is generally a bit higher in the first few months, then it tapers off. But an average churn of 13% leaves just 20% of subscribers crossing that magical 85/15 threshold.
In practice, what this means is that, for all the hype around the 85/15 split, very few developers are going to see a meaningful increase in revenue:
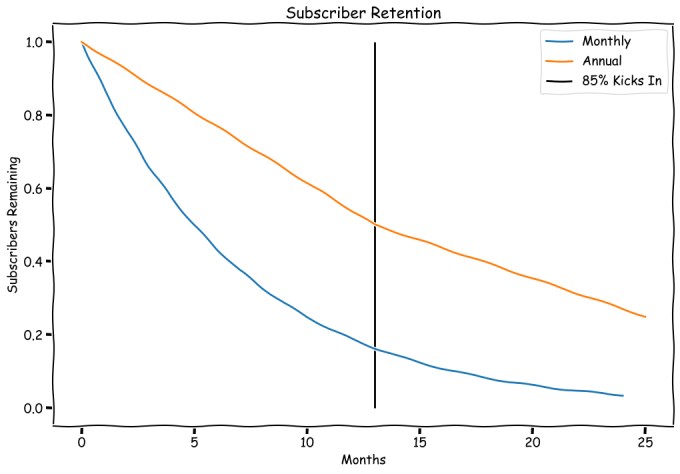
Image Credits: RevenueCat (opens in a new window)
Read Full Article
Improving the Accuracy of Genomic Analysis with DeepVariant 1.0
Posted by Andrew Carroll, Product Lead, and Pi-Chuan Chang, Technical Lead, Google Health
Sequencing genomes involves sampling short pieces of the DNA from the ~6 billion pairs of nucleobases — i.e., adenine (A), thymine (T), guanine (G), and cytosine (C) — we inherit from our parents. Genome sequencing is enabled by two key technologies: DNA sequencers (hardware) that "read" relatively small fragments of DNA, and variant callers (software) that combine the reads to identify where and how an individual's genome differs from a reference genome, like the one assembled in the Human Genome Project. Such variants may be indicators of genetic disorders, such as an elevated risk for breast cancer, pulmonary arterial hypertension, or neurodevelopmental disorders.
In 2017, we released DeepVariant, an open-source tool which identifies genome variants in sequencing data using a convolutional neural network (CNN). The sequencing process begins with a physical sample being sequenced by any of a handful of instruments, depending on the end goal of the sequencing. The raw data, which consists of numerous reads of overlapping fragments of the genome, are then mapped to a reference genome. DeepVariant analyzes these mappings to identify variant locations and distinguish them from sequencing errors.
Soon after it was first published in 2018, DeepVariant underwent a number of updates and improvements, including significant changes to improve accuracy for whole exome sequencing and polymerase chain reaction (PCR) sequencing.
We are now releasing DeepVariant v1.0, which incorporates a large number of improvements for all sequencing types. DeepVariant v1.0 is an improved version of our submission to the PrecisionFDA v2 Truth Challenge, which achieved Best Overall accuracy for 3 of 4 instrument categories. Compared to previous state-of-the-art models, DeepVariant v1.0 significantly reduces the errors for widely-used sequencing data types, including Illumina and Pacific Biosciences. In addition, through a collaboration with the UCSC Genomics Institute, we have also released a model that combines DeepVariant with the UCSC’s PEPPER method, called PEPPER-DeepVariant, which extends coverage to Oxford Nanopore data for the first time.
Sequencing Technologies and DeepVariant
For the last decade, the majority of sequence data were generated using Illumina instruments, which produce short (75-250 bases) and accurate sequences. In recent years, new technologies have become available that can sequence much longer pieces, including Pacific Biosciences, which can produce long and accurate sequences up to ~15,000 bases in length, and Oxford Nanopore, which can produce reads up to 1 million bases long, but with higher error rates. The particular type of sequencing data a researcher might use depends on the ultimate use-case.
Because DeepVariant is a deep learning method, we can quickly re-train it for these new instrument types, ensuring highly accurate sequence identification. Accuracy is important because a missed variant call could mean missing the causal variant for a disorder, while a false positive variant call could lead to identifying an incorrect one. Earlier state-of-the-art methods could reach ~99.1% accuracy (~73,000 errors) on a 35-fold coverage Illumina whole genome, whereas an early version of DeepVariant (v0.10) had ~99.4% accuracy (46,000 errors), corresponding to a 38% error reduction. DeepVariant v1.0 reduces Illumina errors by another ~22% and PacBio errors by another ~52% relative to the last DeepVariant release (v0.10).
DeepVariant Overview
DeepVariant is a convolutional neural network (CNN) that treats the task of identifying genetic variants as an image classification problem. DeepVariant constructs tensors, essentially multi-channel images, where each channel represents an aspect of the sequence, such as the bases in the sequence (called read base), the quality of alignment between different reads (mapping quality), whether a given read supports an alternate allele (read supports variant), etc. It then analyzes these data and outputs three genotype likelihoods, corresponding to how many copies (0, 1, or 2) of a given alternate allele are present.
Technical Improvements in DeepVariant v1.0
Because DeepVariant uses the same codebase for each data type, improvements apply to each of Illumina, PacBio, and Oxford Nanopore. Below, we show the numbers for Illumina and PacBio for two types of small variants: SNPs (single nucleotide polymorphisms, which change a single base without changing sequence length) and INDELs (insertions and deletions).
- Training on an extended truth set
The Genome in a Bottle consortium from the National Institute of Standards and Technology (NIST) creates gold-standard samples with known variants covering the regions of the genome. These are used as labels to train DeepVariant. Using long-read technologies the Genome in a Bottle expanded the set of confident variants, increasing the regions described by the standard set from 85% of the genome to 92% of it. These more difficult regions were already used in training the PacBio models, and including them in the Illumina models reduced errors by 11%. By relaxing the filter for reads of lower mapping quality, we further reduced errors by 4% for Illumina and 13% for PacBio.
- Haplotype sorting of long reads
We inherit one copy of DNA from our mother and another from our father. PacBio and Oxford Nanopore sequences are long enough to separate sequences by parental origin, which is called a haplotype. By providing this information to the neural network, DeepVariant improves its identification of random sequence errors and can better determine whether a variant has a copy from one or both parents.
- Re-aligning reads to the alternate (ALT) allele
DeepVariant uses input sequence fragments that have been aligned to a reference genome. The optimal alignment for variants that include insertions or deletions could be different if the aligner knew they were present. To capture this information, we implemented an additional alignment step relative to the candidate variant. The figure below shows an additional second row where the reads are aligned to the candidate variant, which is a large insertion. You can see sequences that abruptly stop in the first row can now be fully aligned, providing additional information.
- Use a small network to post-process outputs
Variants can have multiple alleles, with a different base inherited from each parent. DeepVariant’s classifier only generates a probability for one potential variant at a time. In previous versions, simple hand-written rules converted the probabilities into a composite call, but these rules failed in some edge cases. In addition, it also separated the way a final call was made from the backpropagation to train the network. By adding a small, fully-connected neural network to the post-processing step, we are able to better handle these tricky multi-allelic cases.
- Adding data to train the release model
The timeframe for the competition was compressed, so we trained only with data similar to the challenge data (PCR-Free NovaSeq) to speed model training. In our production releases, we seek high accuracy for multiple instruments as well as PCR+ preparations. Training with data from these diverse classes helps the model generalize, so our DeepVariant v1.0 release model outperforms the one submitted.
The charts below show the error reduction achieved by each improvement.
![]() |
![]() |
Training a Hybrid model
DeepVariant v1.0 also includes a hybrid model for PacBio and Illumina reads. In this case, the model leverages the strengths of both input types, without needing new logic.
We observed no change in SNP errors, suggesting that PacBio reads are strictly superior for SNP calling. We observed a further 49% reduction in Indel errors relative to the PacBio model, suggesting that the Indel error modes of Illumina and PacBio HiFi can be used in a complementary manner.
PEPPER-Deepvariant: A Pipeline for Oxford Nanopore Data Using DeepVariant
Until the PrecisionFDA competition, a DeepVariant model was not available for Oxford Nanopore data, because the higher base error rate created too many candidates for DeepVariant to classify. We partnered with the UC Santa Cruz Genomics Institute, which has extensive expertise with Nanopore data. They had previously trained a deep learning method called PEPPER, which could narrow down the candidates to a more tractable number. The larger neural network of DeepVariant can then accurately characterize the remaining candidates with a reasonable runtime.
The combined PEPPER-DeepVariant pipeline with the Oxford Nanopore model is open-source and available on GitHub. This pipeline was able to achieve a superior SNP calling accuracy to DeepVariant Illumina on the PrecisionFDA challenge, which is the first time anyone has shown Nanopore outperforming Illumina in this way.
Conclusion
DeepVariant v1.0 isn’t the end of development. We look forward to working with the genomics community to further maximize the value of genomic data to patients and researchers.
MIT engineers develop a totally flat fisheye lens that could make wide-angle cameras easier to produce
Engineers at MIT, in partnership with the University of Massachusetts at Lowell, have devised a way to build a camera lens that avoids the typical spherical curve of ultra-wide-angle glass, while still providing true optical fisheye distortion. The fisheye lens is relatively specialist, producing images that can cover as wide an area as 180 degrees or more, but they can be very costly to produce, and are typically heavy, large lenses that aren’t ideal for use on small cameras like those found on smartphones.
This is the first time that a flat lens has been able to product clear, 180-degree images that cover a true panoramic spread. The engineers were able to make it work by patterning a thin wafer of glass on one side with microscopic, three-dimensional structures that are positioned very precisely in order to scatter any inbound light in precisely the same way that a curved piece of glass would.
The version created by the researchers in this case is actually designed to work specifically with the infrared portion of the light spectrum, but they could also adapt the design to work with visible light, they say. Whether IR or visible light, there are a range of potential uses of this technology, since capturing a 180-degree panorama is useful not only in some types of photography, but also for practical applications like medical imaging, and in computer vision applications where range is important to interpreting imaging data.
This design is just one example of what’s called a ‘Metalens’ – lenses that make use of microscopic features to change their optical characteristics in ways that would traditionally have been accomplished through macro design changes – like building a lens with an outward curve, for instance, or stacking multiple pieces of glass with different curvatures to achieve a desired field of view.
What’s unusual here is that the ability to accomplish a clear, detailed and accurate 180-degree panoramic image with a perfectly flat metalens design came as a surprise even to the engineers who worked on the project. It’s definitely an advancement of the science that goes beyond what may assumed was the state of the art.
Read Full Article